
I can’t resist blogging about the performance results we’re seeing for the Hydroflow dataflow runtime. This is a Rust-based library that is part of the Hydro project (more background below!)
You can think about Hydroflow as a single-node dataflow library, kind of like Spark or Pandas, written in Rust. Except that (a) Hydroflow is tuned for extremely low-latency handling of data, (b) it has networking functionality so that many Hydroflow programs can work in concert as a distributed system, and (c) it has semantic properties that make it amenable to high-level distributed optimizations and correctness analysis. The intended use case for Hydroflow is to be a runtime kernel for high-performance distributed systems.
And perform it does! Using Hydroflow, we have recently been able to get some world-beating performance numbers for classic distributed systems challenge problems. These are rough numbers, not scholarly results, but provide strong evidence that Hydroflow is as fast or faster than handwritten code in languages like C++ and Scala, despite being a higher-level language that is is amenable to many kinds of distributed optimizations and program checks in future.
Meanwhile, on to the numbers!
Case Study #1: Compartmentalized Paxos
A few years back, the inimitable Michael Whittaker was doing his Ph.D. at Berkeley. A serious student of distributed protocols, he observed that the godparent of consensus—Multipaxos—could be scaled up with simple but elegant techniques he called “compartmentalization”. Using these simple techniques he was able to build an implementation of MultiPaxos whose throughput scaled better than many much more complex schemes in the literature. All this is documented in his VLDB 2021 paper on Compartmentalized Paxos. Michael implemented Compartmentalized Paxos by hand in Scala.
The Hydro team recently reimplemented Michael’s scheme in a high-level spatiotemporal logic language called Dedalus, which compiles down to Hydroflow, which in turn is a Rust library that is compiled by llvm. And here’s the results to the right. The curves to focus on are the ones on the far right, which are giving huge throughput at low latency. “CScalablePaxos” is Michael Whittaker’s Scala code; “DedalusCScalablePaxos” is our Dedalus implementation of Michael’s design. All this is running on GCP using 20 n2-standard-4 machines
with 4 vCPUs, 16 GB RAM, and 10 Gbps network bandwidth, with one machine per node. Bottom line: Hydroflow’s performance on Compartmentalized Paxos is a bit better than Michael Whittaker’s state-of-the-art (as of 2021) handwritten Scala code. (The other curves are experimental results that will hopefully get explained in an upcoming paper.)
Case Study #2: Anna KVS
Readers of this blog will be familiar with the Anna Key-Value Store database. Anna was designed to run coordination-free across cores and machines. Upon first publication, it was especially good at high-contention workloads that other systems would fail at–it was up to 700x faster than Masstree, up to 800x Intel’s “lock-free” TBB hash table. Mind you it didn’t provide serializable consistency, but it did provide many coordination-free consistency levels, including Causal Consistency and Read Committed isolation. For our purposes here though, the question is whether a Hydroflow implementation can compete with Anna’s native C++ implementation.
Unlike Compartmentalized Paxos, the original experimental harness for Anna is lost in the mists of time and git. As a result it’s hard to recreate the graphs from the original Anna paper as we did for compartmentalized Paxos. The best we can do is show the 5-year-old Anna graph next to a graph of a Hydroflow implementation on the same workload using similar machines. And here are the results! The first, colorful graph is from the original Anna paper; the second shows new results from Hydroflow.
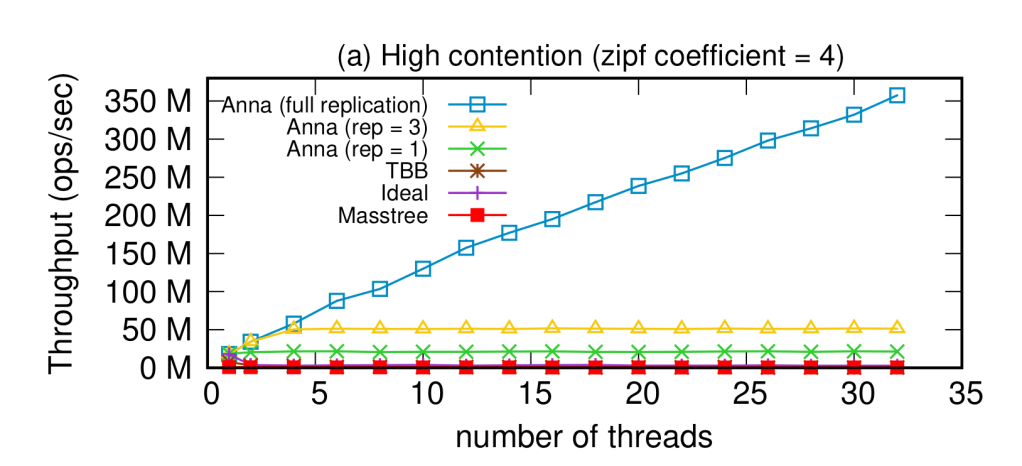
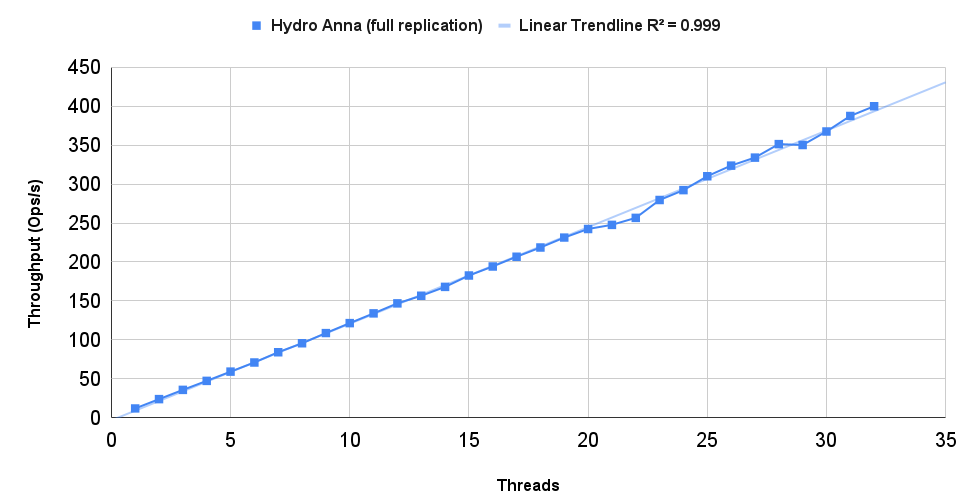
Note the sweet linear scaling in both cases — that’s thanks to Anna’s coordination-free design. Note also that the Hydroflow implementation’s throughput is in the same order of magnitude as C++ Anna, i.e. 2+ orders of magnitude faster than Masstree and TBB. Mind you the original numbers in the first chart are from Amazon m4.16xlarge instances (64 vCPU and 256GB RAM) in 2018, and the Hydroflow numbers are from GCP n2-standard-64 instances (64 vCPU, 256GB RAM) in 2023. So while I won’t claim that Hydroflow is “faster” than the C++ codebase, it’s very clearly in the ballpark and shows evidence of being about as fast as handwritten C++.
HT to Lucky Katahanas and Mingwei Samuel, the primary developers of Hydroflow, as well as David Chu, Chris Liu, Shadaj Laddad, Rithvik Panchapakesan who all contributed to the Compartmentalized Paxos implementation. (A modest fraction of Hydroflow code was also written by yours truly.)
Background on Hydro
The Hydro project is a multi-year effort at UC Berkeley and Sutter Hill Ventures. The Hydro Project is developing cloud-native programming models that allow anyone to develop scalable and resilient distributed applications that take full advantage of cloud elasticity.
At the time of writing, Hydroflow is maturing into a high-performance open source runtime for low-latency dataflow. Hydroflow is designed with two use cases in mind:
- Expert developers can program Hydroflow directly to build components in a distributed system.
- Higher levels of the Hydro stack will offer friendlier languages with more abstractions, and treat Hydroflow as a compiler target.
Hydroflow provides a DSL—a surface syntax—embedded in Rust, which compiles to high-efficiency machine code. As the lowest level of the Hydro stack, Hydroflow requires some knowledge of Rust to use. Hydroflow is well-documented online, and via its source code on github.
We laid out our vision for Hydro in a paper at CIDR 2021 called “New Directions in Cloud Programming”. In that paper we proposed a compiler stack with multiple components, as shown to the right. At the highest level of input, we hope to support a multitude of distributed programming styles, much like LLVM provides a compiler stack for a multitude of sequential programming languages. Also like LLVM, Hydro envisions multiple layers of intermediate representation languages (IRs) that can serve as common ground for program checks and transformations, providing developers with various entry points to work deeper in the stack as they see fit. The top layer of Hydro we call Hydraulic: a system to lift low-level code from legacy interfaces into a higher-level declarative distributed IR we dub Hydrologic. The next layer down is an optimizing compiler we call Hydrolysis, which takes Hydrologic and compiles it to run on multiple instances of a single-threaded, asynchronous dataflow IR called Hydroflow.
Want to learn more? Check out our webpage at https://hydro.run.